Oops, it has been such a long time since I wrote a post that I even accidentally forgot to renew my site! That’s bad. I definitely owe you a few new posts. Thus, here is another post in the distributed systems magic series.
Recently I undertook the task of improving the proxying logic in Thanos. If you are not familiar with it, the Query component of Thanos sends out requests via gRPC to leaf nodes to retrieve needed data when it gets some kind of PromQL query from users. This article will show you how it worked previously, how it works now, and the learnings from doing this.
It assumes some level of familiarity with Go, Thanos, and Prometheus.
How Proxying Logic Works Currently (v0.28.0 and before)
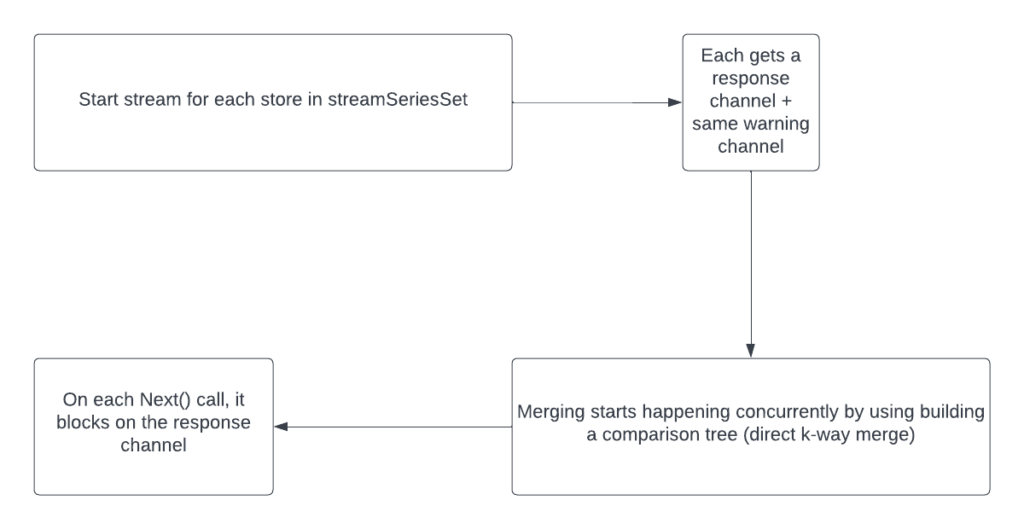
Since it compares each node with every other node, the complexity of this is Θ(kn):
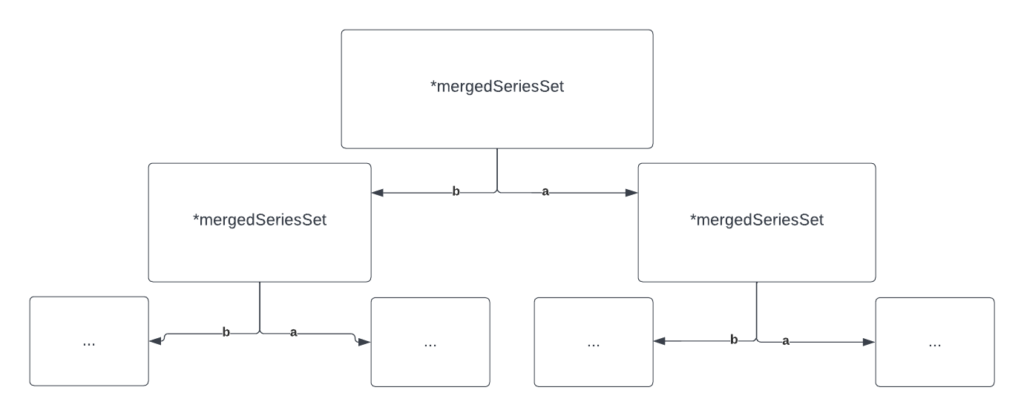
We can improve by first of all using a different data structure. You can easily see that a direct k-way merge is not the most efficient one – it is unnecessary to compare everything all the time. Another thing is that *mergedSeriesSet
uses a buffered Go channel inside which means that we cannot receive messages from leaf nodes as quickly as possible. Let’s fix this.
How Proxying Logic Works Now (v0.29.0 and after)
First of all, let’s start using a data structure that allows doing k-way merge much faster. There are different options here but since Go has a pretty good heap container structure already in the standard library, we’ve decided to use that. With this, we have moved to logarithmic complexity: O(nlogk) instead of Θ(kn).
Another major performance bottleneck is the usage of a channel. I vaguely remember a meme on Twitter that went something along these lines: “Go programmers: use channels. Performance matters? Don’t use channels”. In the new version, the proxying logic uses time.Timer to implement cancelation on a Recv()
(a function that blocks until a new message is available in a gRPC stream) that takes too long. Please see this awesome post by Povilas to get to know more information about timeouts in Thanos. This means that channels are no longer needed.
Finally, there has been a long-standing problem with the PromQL engine that it is not lazy. What this means is that ideally all of the retrieved data should be directly passed to the PromQL engine as fast as possible while it iterates through series & steps. However, right now everything is buffered in memory, and the query is executed only then. Hence, this refactoring should also allow us to easily switch between lazy and eager logic in the proxying layer.
Here is a graphic showing the final result:
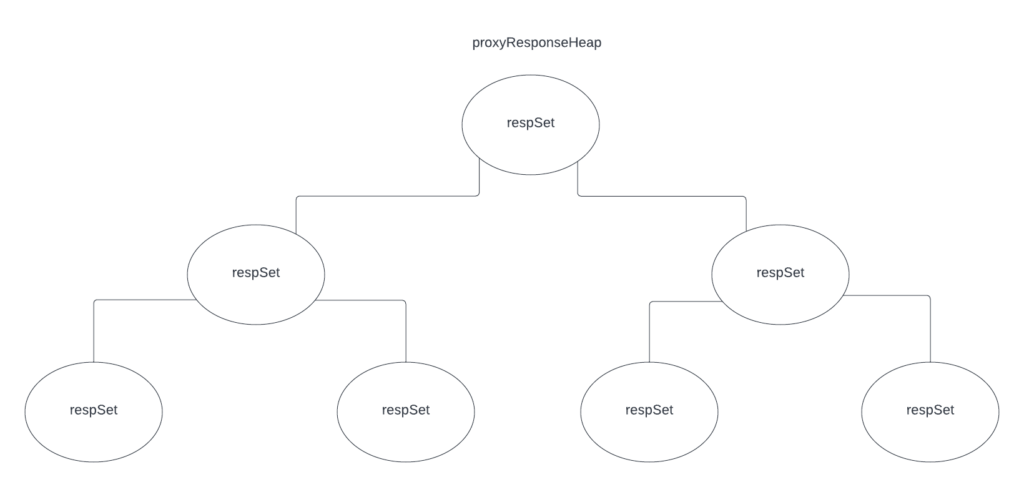
respSet
is a container of responses that can either be lazily retrieved or eagerly. dedupResponseHeap
is for implementing the heap interface. In this way, the retrieval strategy is hidden in the container and now the code is tidy because the upper heap container does not care about those things.
You can see that visually this looks like the previous graphic however we are now actually taking proper advantage of the tree-like structure.
Learnings
I have learned lots of things while implementing this.
First of all, looking at something with fresh eyes and lots of experience maintaining code brings a new perspective. I remember someone saying that if after a year or two you can still look at your old code and don’t see much or any potential improvements then you aren’t really advancing as a developer. I reckon that it is kind of true. Same as in this case – after some time and after some thinking it is easy to spot improvements that could be made.
Another thing is that benchmarks are sometimes not valued as much. Go has very nice tooling for implementing benchmarks. We should write benchmarks more often. However, my own personal experience shows that companies or teams usually focus on new features and benchmarks/performance becomes a low-priority item for them. My friend Bartek is working on a book Efficient Go that has some great information and learnings about benchmarks. I recommend reading it once it comes out.
Finally, I think all of this shows that sometimes bottlenecks can be in unexpected places. In this case, one of the bottlenecks was hidden deep inside of a struct’s method – a channel was used for implementing per-operation timeouts. This is another argument in favor of benchmarking.
Now, with all of this, the proxying layer is still not fully lazy because there are wrappers around it that buffer responses however that is for another post. With all of the improvements outlined in this post, it should be quite trivial to fully stream responses.
Thanks to Filip Petkovski and Bartek Plotka for reviewing & testing!