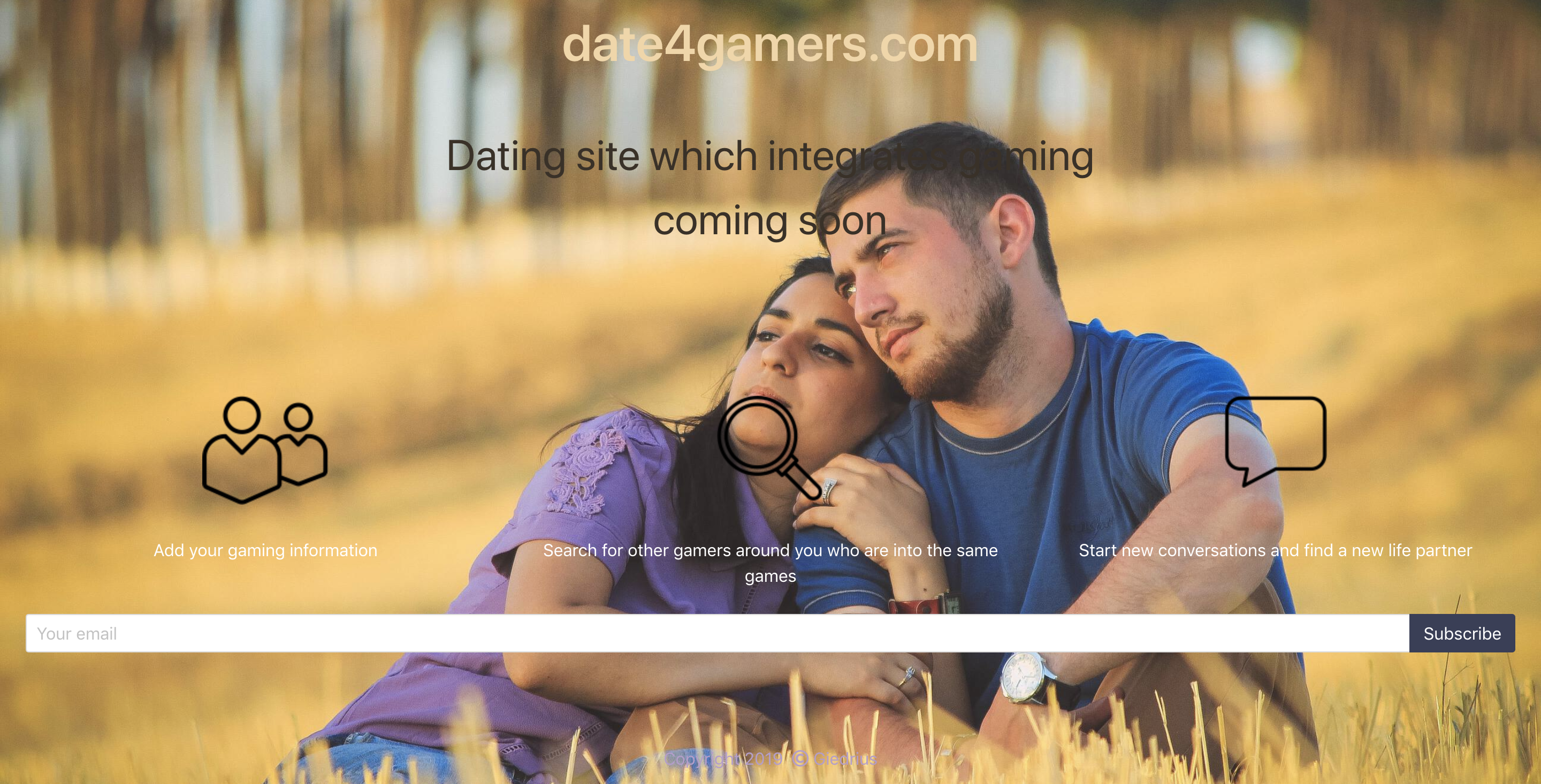
Like almost everyone, I also dream about starting my own business so that I could be free from the shackles of someone else and I would be my own boss. Or it could become potentially a source of passive income.
As such, I have started reading some literature and sites like Indiehackers to learn about how others start their own software businesses. After all, software is the thing that I am most skilled in and so I ought to connect that with the other things which are involved in having a successful business to start my own software company.
This will be a post about my attempt to validate the first product idea. The whole purpose of that is to check if your idea is viable i.e. it solves actual problems that people have, if it is feasible, before actually starting to build it.
Idea
I thought there was a place in the market for a dating site which would connect two different things – gaming and dating. The dating site would have provided a way to add more info about yourself besides games. Originally, it should have only supported Steam so that you could, essentially, find people around you who are into the same games.
Furthermore, it would have had Tinder-style dating – essentially it would have used a “minimalistic” user interface through which one could’ve been matched with other people who were playing the same type of games, and or the same amount of time.
Timeline
Initial problems
Having or making a dating already entails a lot of issues:
- the privacy of its users as per the GDPR and what the users expect – the ability to request information about yourself that you have in the system, the ability to delete your own account, and so on;
- protection against harassment and perils.
Thus, it means that if one were to make a prototype dating site, it would take so much more time to bring it up to a level which was necessary for any kind of website like that. That’s why I have chosen to make a landing page at first.
Landing page
I made the website with simple static HTML and JS, and by using the Bulma CSS framework. I have used this template as a reference. Let me confess: at first I have tried to do a landing page without using any kind of CSS framework but in $CURRENT_YEAR it is nigh impossible to do that and have the website scale to all kinds of different devices effortlessly. I had some kind prototype version that uses pure CSS but when I had opened it on my Samsung phone, I saw a horrible misrendering of it.
The value proposition to the potential users should be clear from the landing page but it was kind of hard to do that in my case. However, I agree that I could have done a better job – it is kind of hard to understand how my website was to differentiate from others judging just from that landing page. On the other hand, I think that there wouldn’t have been much difference because we already know now in hindsight that it is an oversaturated market already, it is hard to achieve a breakthrough, and that this is not a problem that the majority of the people who use dating sites have.
Also, you can tell from the design that I am not the best at it – my brain is trained to care much more about the functional properties of things instead of the design – ease of use, understanding, attracting users. I still need to improve a lot on this. That’s why I am thinking that for the next attempt I will create a prototype which will not have a lot of user interface elements, and it will be mostly a service which provides value for its users.
Facebook woes
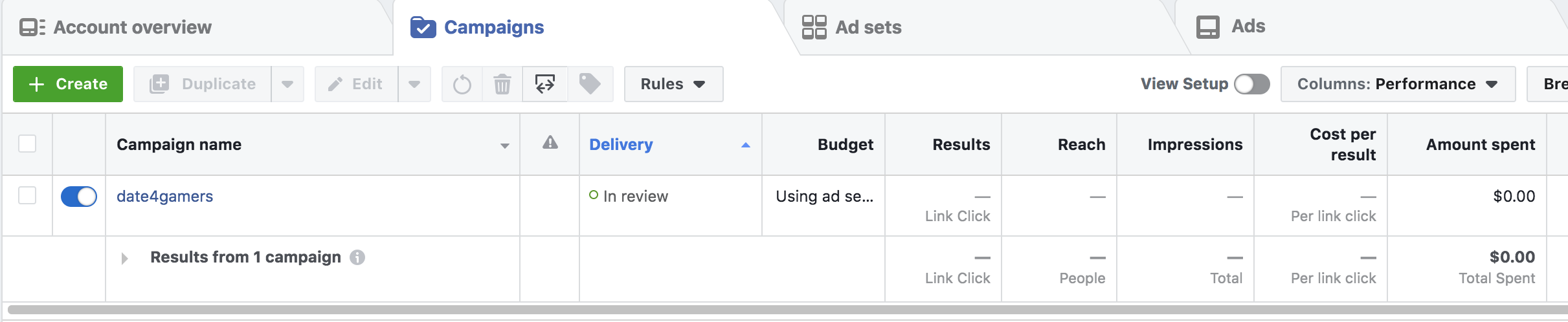
At first I wanted to make my campaign on Facebook but funnily enough, they do not even accept advertisement campaigns which have anything to do with dating. This is most certainly related to my points before – it is hard to make a good-enough dating site. Even a prototype.
Also, Facebook’s advertisement campaigns are a bit of a pain in the ass since you have to create an associated page in their system with the ad – probably because people can see which page has released that by clicking on the burger menu.
After all of this, I have decided to go to Google’s Ads.
How did it go
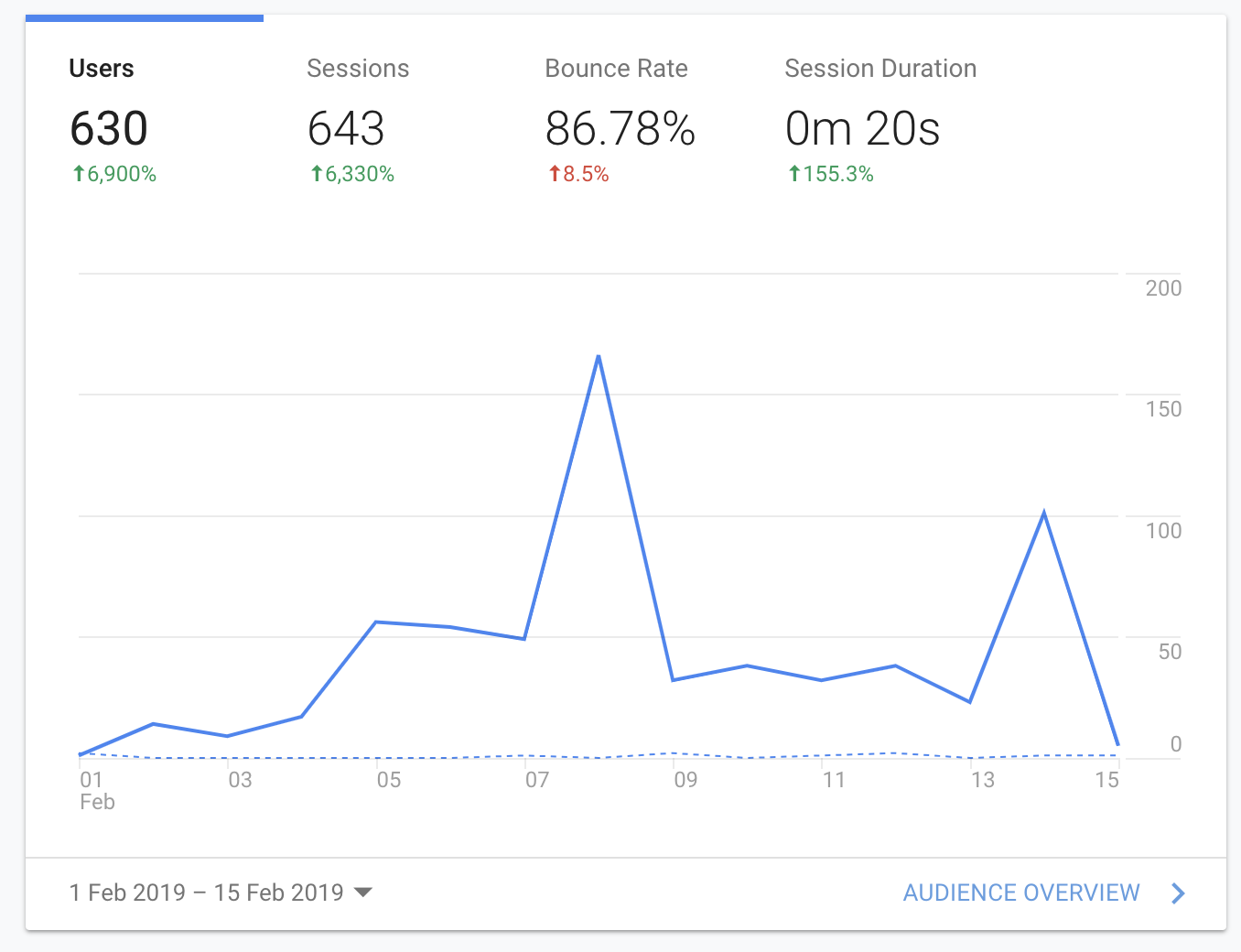
I have spent 20 euros on this advertisement campaign and I got around 630 users are you can see in this picture. Only 2 users have signed up to the mailing list which means that I got a very minuscule 0.3% of conversions.
This indeed spells out a very negative response to the landing page and the whole idea. However, perhaps my campaign was not as effective since it seems like the majority of people came from countries where English is not an official language.
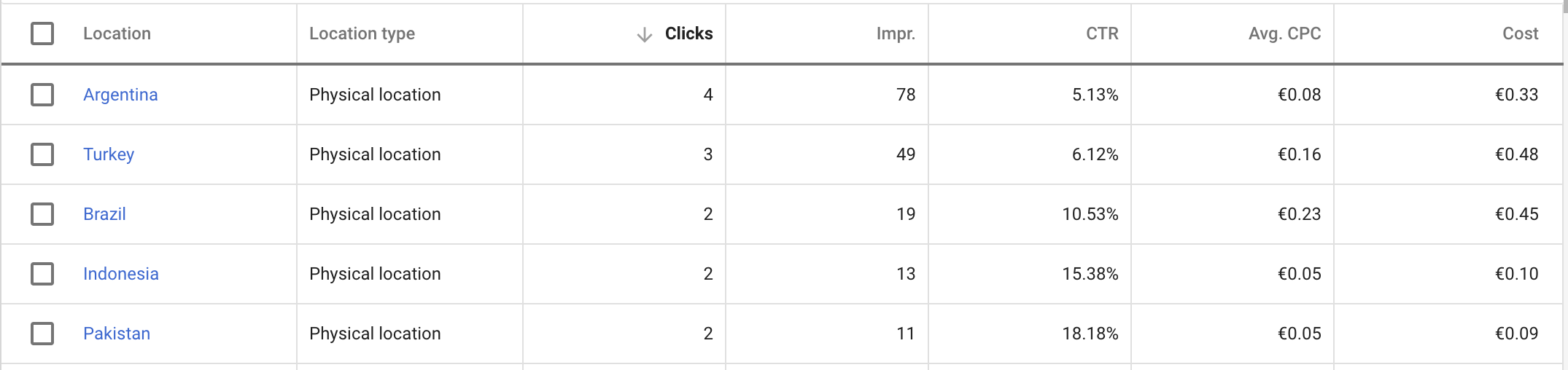
Funnily enough, the people who registered for the mail campaign are from India and Saudi Arabia. I want to say that perhaps this can be associated with the state of the society in those countries i.e. repression of women’s rights, and the general gender disbalance there? I don’t actually know but just with this data, I think, we can tell that the market for this kind of thing is simply not big enough.
Conclusion
Any kind of product idea that you might have when presented to others should immediately attract an immense amount of potential clients. If not, then it’s most likely not worth doing like in my case. Also, ideally you would have some kind of prototype to show to users so that you could attract them even more. A picture is worth a thousand words but a working prototype (the MVP, maybe even) is worth a thousand pictures because it allows the users to get a feel of it and make their own opinion about it.
Certain types of ideas are very risky such as dating sites because they are associated with scammers who use sex as a way to bait others into visiting their website, and sending their own bank account details.
In foresight, it might be hard to tell where your potential customers are if you are targeting a wide audience. That is why ideally you should work with concrete people who have specific problems that you should try to solve.
And I will try to soak up all of these lessons for the next side project attempt that I am going to do in the near future, as should you.